My work centers on advancing the understanding of atmospheric chemistry and its intersections with human and environmental systems. I am particularly interested in leveraging innovative data science and machine learning techniques to address complex challenges in air quality and wildfire land/smoke management. I enjoy approaching problems from unique perspectives, uncovering insights through the exploration of unconventional methods and data sources.
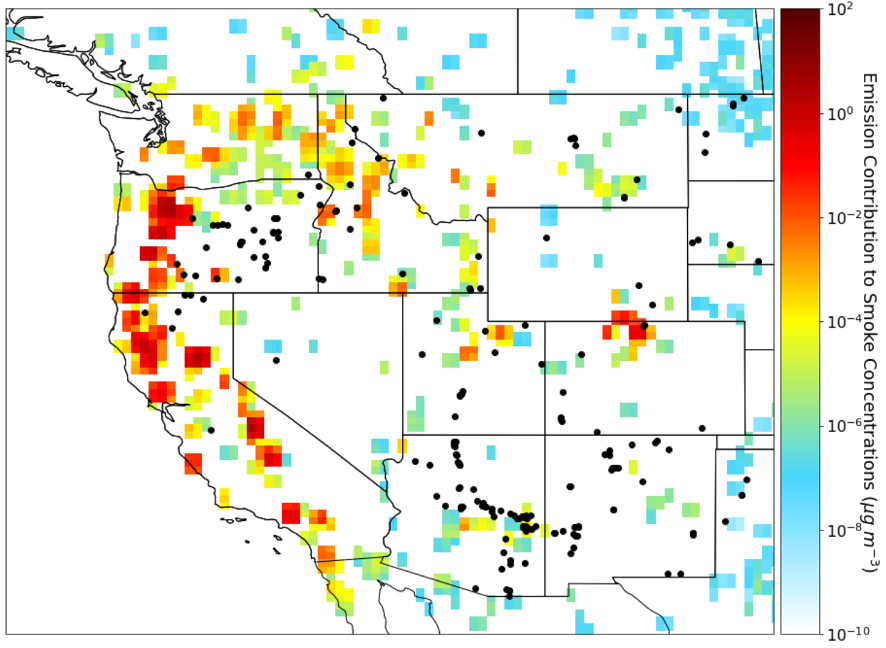
Wildfire, Prescribed Fire Smoke Modeling and Mitigation
Catastrophic wildfires pose substantial risk to public health, infrastructures, and ecosystems in the western United States. As these large and costly wildfires become more common, methods to identify locations for prescribed burning are needed to mitigate impacts on affected populations and ecosystems. Here we investigate the effectiveness of prescribed burning for abating potential wildfire smoke exposures in states and rural environmental justice communities across the western United States during the destructive 2018 and 2020 fire seasons. We find that due in part to prevailing wind patterns, wildfires in the coastal states contribute more to overall smoke exposure in the West compared to wildfires in other states in both 2018 and 2020. We show that implementing prescribed burns in the heavily forested Northern California and the Pacific Northwest would yield large net benefits for the entire western United States, while doing so in other states would have relatively smaller impacts. Our work suggests that land managers should prioritize northern California, western Oregon, and eastern Washington for prescribed burns to mitigate future smoke exposure as these regions have a disproportionate impact on smoke exposure for rural environmental justice communities and population centers across the West.
Publications: Kelp et al., (2023) Earth's Future
Co-Authored Wildfire Publications: Qiu et al., (2024), Liu et al., (2024)
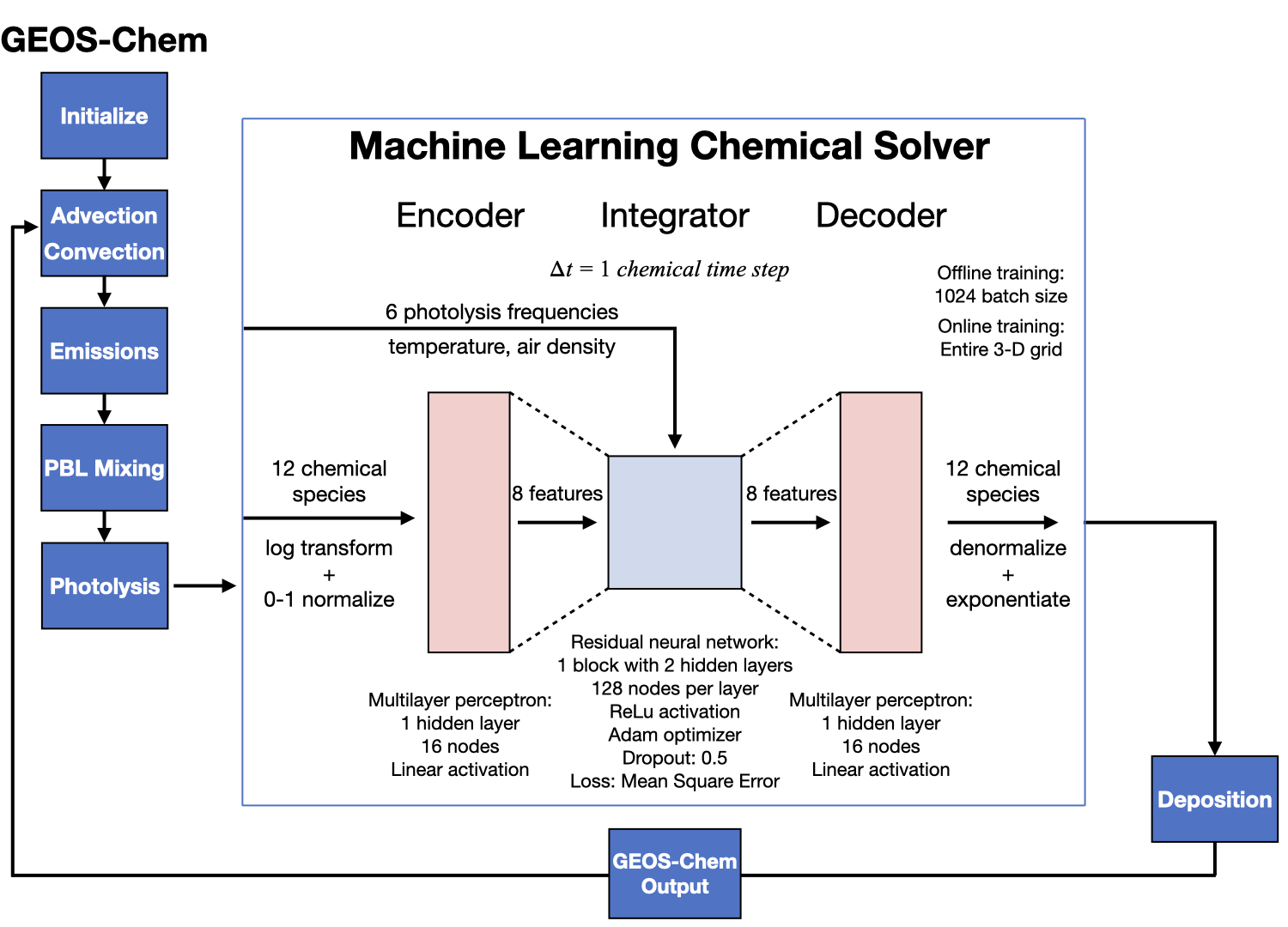
Deep Learning Atmospheric Chemistry
Global models of atmospheric chemistry are computationally expensive. The chemical solver that integrates the large-dimensional coupled systems of kinetic equations describing the chemical mechanism is a bottleneck. Machine learning (ML) could be transformative for reducing the cost of an atmospheric chemistry simulation by replacing the chemical solver with a faster emulator. However, my past work found that ML chemical solvers experience rapid error growth and become unstable over time. My current work aims to achieve, for the first time, a stable full-year global simulation of atmospheric chemistry with three-month seasonal ML solvers. We show that online training of the ML solver synchronously with an atmospheric chemistry model simulation produces considerably more stable results than offline training from a static dataset of simulation results. Although our work represents an important step for using ML solvers in global atmospheric chemistry models, more work is needed to extend it to large chemical mechanisms and to reduce errors during long-term chemical aging.
Publications: Kelp et al., (2022) JAMES, Kelp et al., (2020) JGR: Atmospheres, Kelp et al., (2018) ArXiv
Co-Authored ML Publications: Balasus et al., (2023)
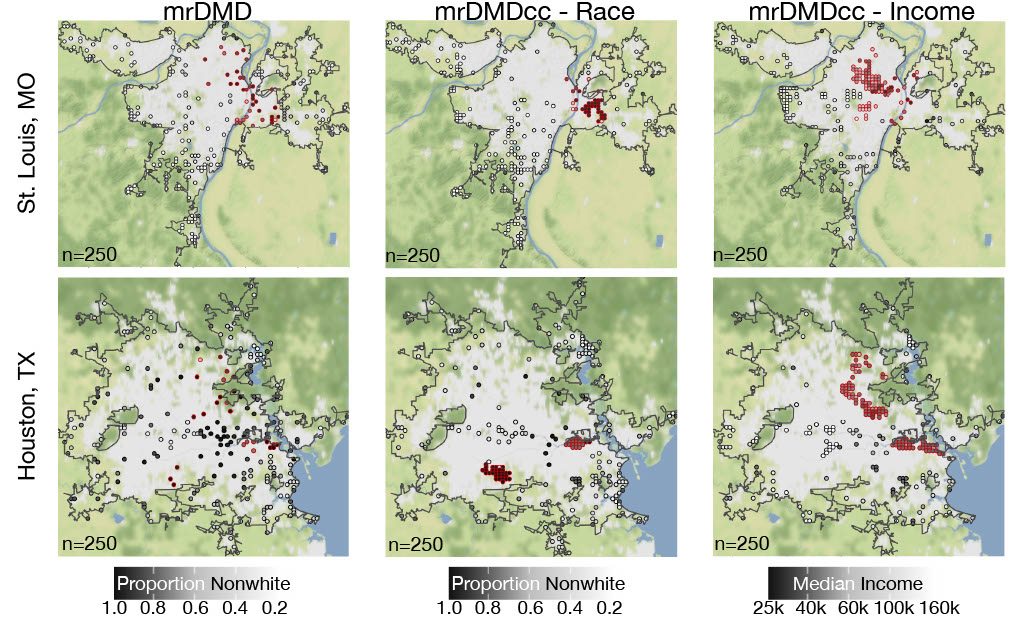
Data-Driven Air Pollution Sensing
In the United States, citizens and policymakers rely upon Environmental Protection Agency (EPA) mandated regulatory networks to monitor air pollution; increasingly, low-cost sensor networks supplement spatial gaps in the regulatory monitor networks. Although these regulatory and low-cost networks provide enhanced spatiotemporal coverage in urban areas, sensors are located most often in higher income, predominantly White areas. Here we use a modal decomposition algorithm to identify the optimal and equitable placement of fine particulate matter (PM2.5) sensors in four U.S. cities with histories of racial or income segregation: St. Louis, Houston, Boston, and Buffalo. Compared to networks using air pollution information alone, the algorithm places a greater number of sensors in historically low-income and nonwhite neighborhoods with known environmental pollution problems, while also capturing PM2.5 extremes. Our work provides a roadmap for the creation of equitable sensor networks in U.S. cities and offers a guide for democratizing air pollution data by increasing spatial coverage of low-cost sensors in less privileged communities.
Publications: Kelp et al., (2023) GeoHealth, Kelp et al., (2022) ERL
Co-Authored Sensor/EJ Publications: Kawano et al., (2025), Yang et al., (2022)
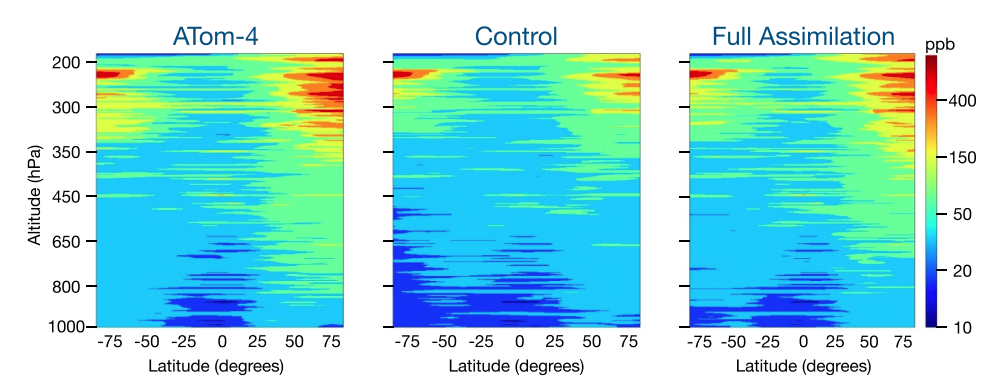
Chemical Data Assimilation for Atmospheric Composition
The NASA Goddard Earth Observing System Composition Forecast system (GEOS-CF) provides global near-real-time analyses and forecasts of atmospheric composition. The current version of GEOS-CF builds on the GEOS general circulation model with Forward Processing assimilation of meteorological data (GEOS-FP) and includes detailed GEOS-Chem tropospheric and stratospheric chemistry. Here we add 3D variational data assimilation in GEOS-CF to assimilate satellite observations of ozone including MLS vertical profiles, OMI total columns, and AIRS and IASI hyperspectral 9.6 μm radiances. We find that the detailed tropospheric chemistry in GEOS-CF significantly improves the simulated background ozone fields relative to previous versions of the GEOS model, allowing for specification of smaller background errors in assimilation and resulting in smaller assimilation increments to correct the simulated ozone. Comparisons to independent ozonesonde and aircraft (ATom-4) observations for 2018 show significant GEOS-CF improvement from the assimilation, particularly in the extratropical upper troposphere.
Publications: Kelp et al., (2023) ERL
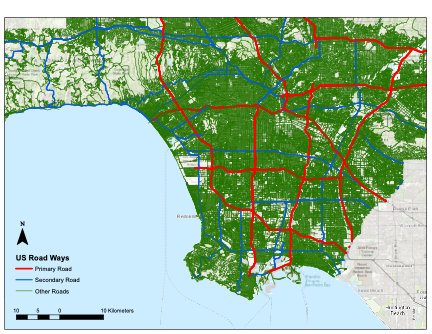
Vehicle Emission Factors for Area-Wide Mobile Monitoring
On-road vehicle emissions are a significant source of outdoor air pollution, which pose a severe human health risk, especially for those who live near busy roads. A city's vehicle fleet can determine the levels of risk and exposure for residences as emissions differ for gasoline- vs. diesel-powered vehicles. Most research sampling mobile source emissions in urban traffic involves “vehicle chase” studies of exhaust plumes from individual vehicles which may not be representative of the average emissions for a given area.
We create a statistical model from measurements obtained from continuously moving platforms to estimate area-wide average vehicle emission factors of neighborhoods. These model predictions are used to estimate emission factors by source-related features within a city. Furthermore, our model can calculate separately light-duty and heavy-duty vehicle emission factors for a study area while also separating out high-emitter vehicles that may artificially skew emission factor estimates. Study areas include Los Angeles, USA and Chengdu, China.
Publications: Kelp et al., (2020) Atmos. Env.
Related Publications: Wen et al., (2019)
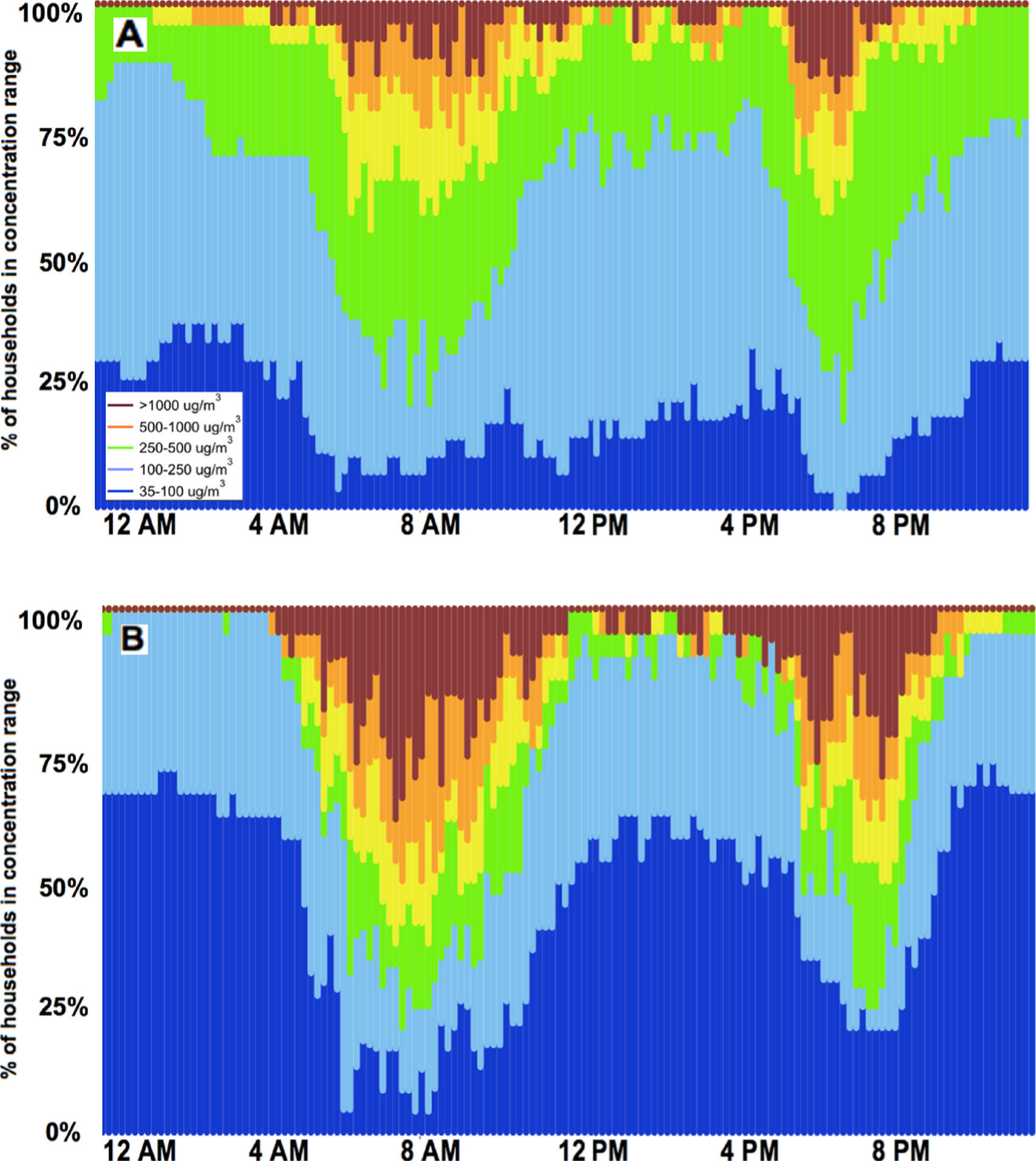
Indoor Air Pollution from Cookstove Interventions in S. India
Biomass combustion from residential cookstoves is a major source of indoor air pollution and a large contributor to the global burden of disease. Investment of resources into rural energy intervention programs has great potential to improve household air quality in developing countries and thus increase quality of life and improve public health. We conducted a randomized intervention study to evaluate air pollution impacts of a carbon-finance-approved cookstove in rural South India. We employed real-time monitors to measure indoor concentrations of PM2.5, black carbon (BC) and carbon monoxide (CO) in households using carbon-finance-approved stoves and households continuing to use traditional open fire stoves. Implementation of the new cookstoves decreased concentrations of CO and PM2.5, but increased BC concentrations relative to the traditional stoves.
Although lab studies have clearly demonstrated the potential benefits of cookstove interventions, achieving these same benefits in real households is more complex. This work suggests that reduction in indoor pollution from intervention cookstoves might not be occurring in practice to the same extent as is expected from lab evaluation, and that benefits from such interventions should not be assumed.
Publications: Kelp et al., (2018)